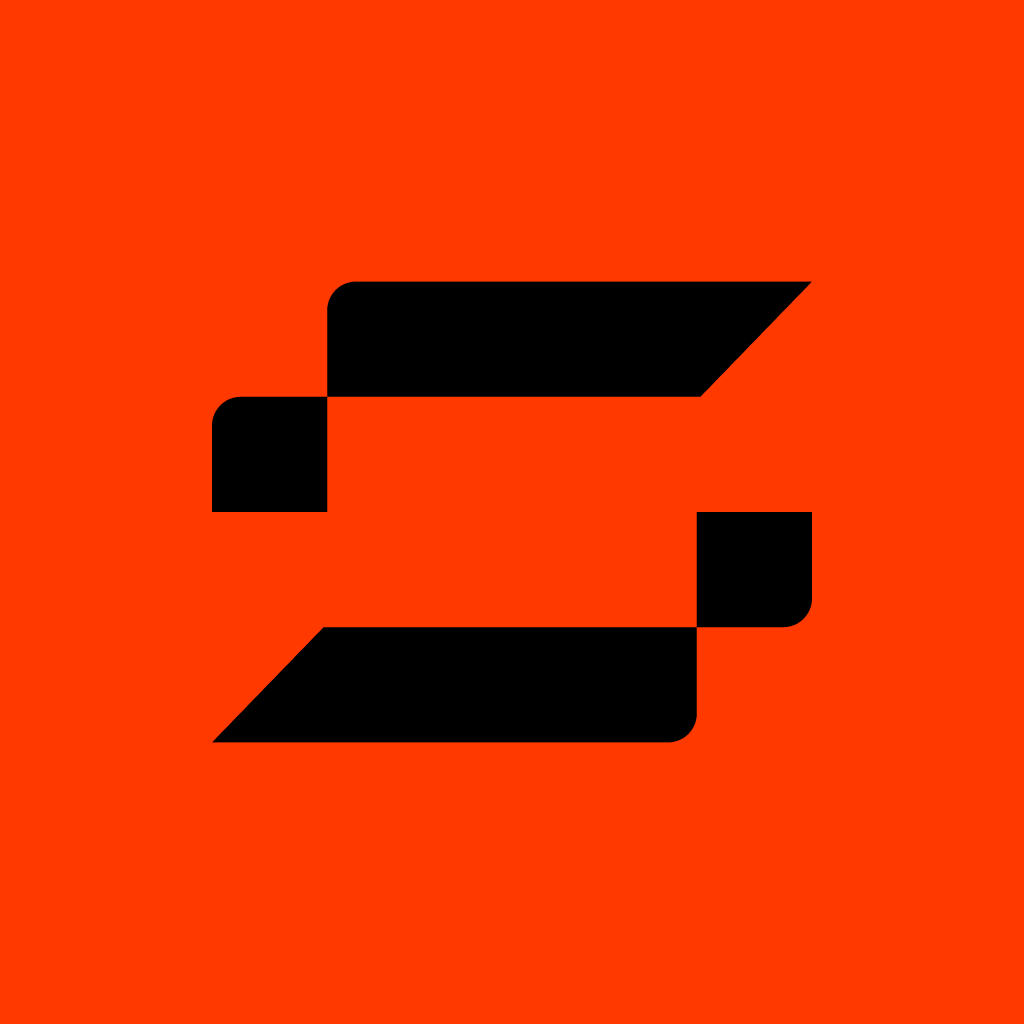
As many of you know, I am quite fond of digging deep into corporate financials. However, there is a physical limit to how much a person can cover. Furthermore, it requires a long time and experience to not miss the nuances of increasingly complex financial reporting documents. Add to this an increasingly complex market and macro environment, and you can quickly see how the skills of a decent credit analyst take quite some time to transfer in a world where timing is crucial considering how fast things evolve nowadays.
In fact, despite the evolution in technology that brought us the Internet first and the #blockchain a decade ago, credit analysis did not do a good job of keeping pace with the speed of financial markets’ evolution. The reason is ultimately very simple: everything that is based on physical human input to achieve an output is very hard to scale. Furthermore, by definition, humans are very subject to being influenced by biases and narratives ultimately setting their mind to what they want to achieve first and then looking for what supports their thesis instead of objectively analyzing all available elements to then come to a solid conclusion. Credit analysis is one of those fields where not keeping human biases can be dangerous and ultimately lead to mistakes that influence important decisions. The article “Credit scores and the bias behind them” provides very good examples of what I am talking about.
Now that blockchain technology is available, potentially enabling far greater transparency and reliability of data than what the internet can provide, and we have the hardware to start running Artificial Intelligence models at scale and in a cost-effective manner, why continue to be stuck with methods that did not evolve that much in the past 50 years with the real major difference of analysis being performed in “Excel spreadsheets” rather than on paper? Exactly, it’s time to evolve and this is why we are building Synnax.
Let’s have a look at this example from the Web3 space here.
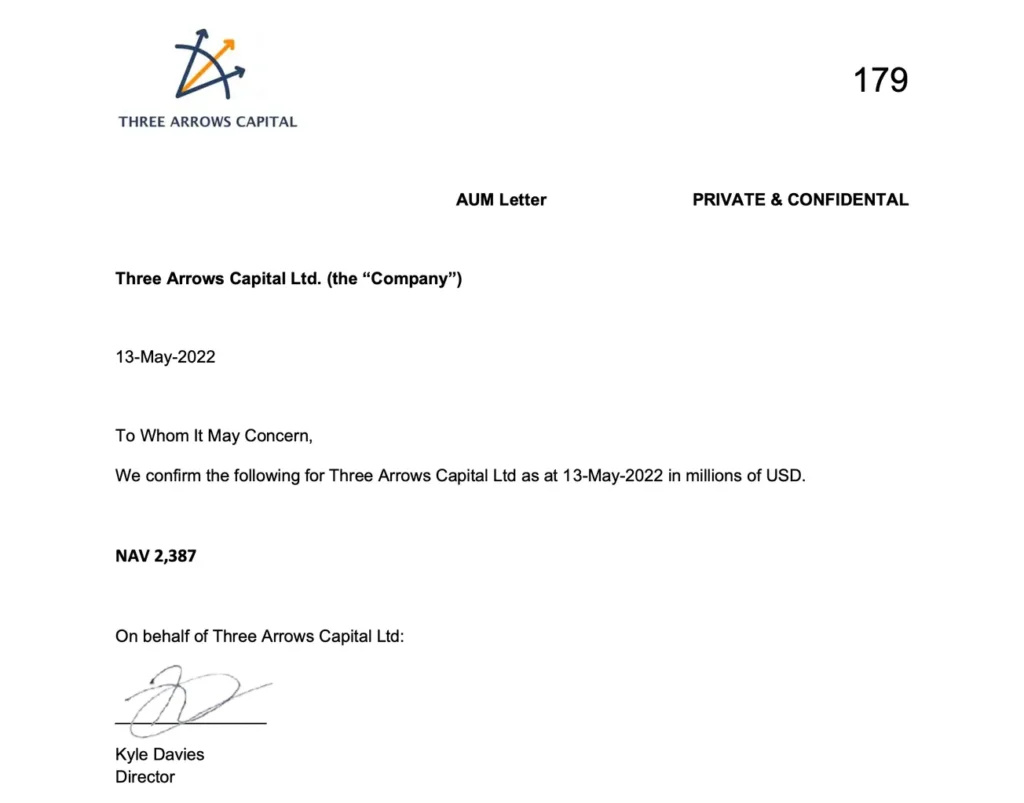
Anyone active in the Web3 space knows how the Three Arrows Capital (3AC) story ended (in case you don’t, this link here has a quick recap), but the real problem is that almost everyone was blindsided by how the events unfolded starting from many of their lending counterparts and trading partners.
How could #Synnax have anticipated what happened and spared a great deal of damage to stakeholders of 3AC?
1 – Synnax is based on a decentralized network of Data Scientists developing machine learning (ML) models in order to predict the financial strength of a company in real-time. Models don’t care about “trust me” documents, all they care about is data. In the case of 3AC, the models would have immediately started to pick up anomalies between the real-time value of assets and liabilities associated with 3AC and the NAV publicly declared by the company.
2 – With the evolution of the situation, through Synnax’s granular Credit Intelligence and forward-looking probability predictions gathered from the decentralized ML models networks, lenders and trading counterparts would have had real-time information available to start questioning the figures being shared by the company. Let me give you an example:
- 3AC had a very large position in the popular “GBTC Arbitrage” trade.
- This trade consisted of buying Bitcoin at X price and then delivering them to Greyscale which was in return creating new units of the trust for equivalent value. Where was the arbitrage? #GBTC traded at a significant premium in the traditional markets compared to #Bitcoin.
-
All 3AC had to do to make a decent profit was to sell the GBTC units they received. Then why did things go south for 3AC?
- 3AC did not purchase the BTC they tendered into GBTC, but they either borrowed them or borrowed money to purchase them.
- 3AC did not sell the GBTC units but used them as collateral to borrow even more funds to inject into this “arbitrage” trade.
3 – Now tell me, what happens to an “arbitrage” when more and more people start exploiting it? It disappears by definition and the GBTC vs BTC one was no exception to it as you can see in the chart below.
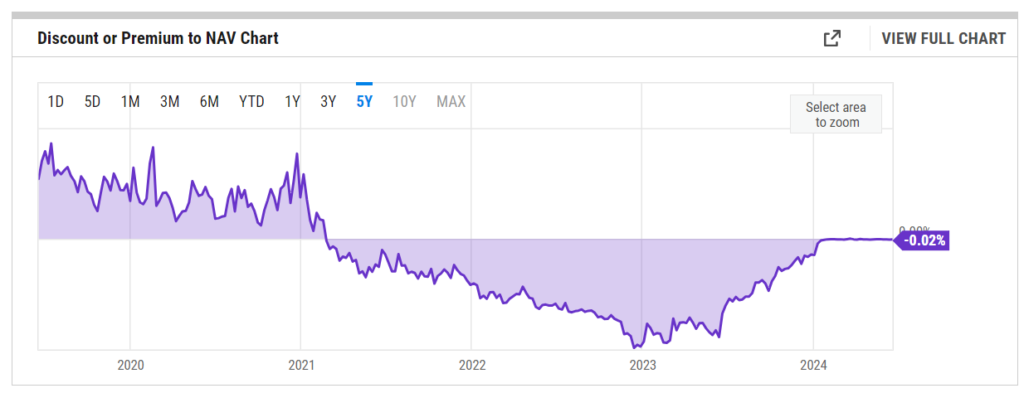
Hold on a second, then why in 2021 did the spread collapse to become negative? As I highlighted before, 3AC (and many other companies) used GBTC as collateral to borrow more funds, keep that in mind and look at the GBTC price vs BTC chart now.
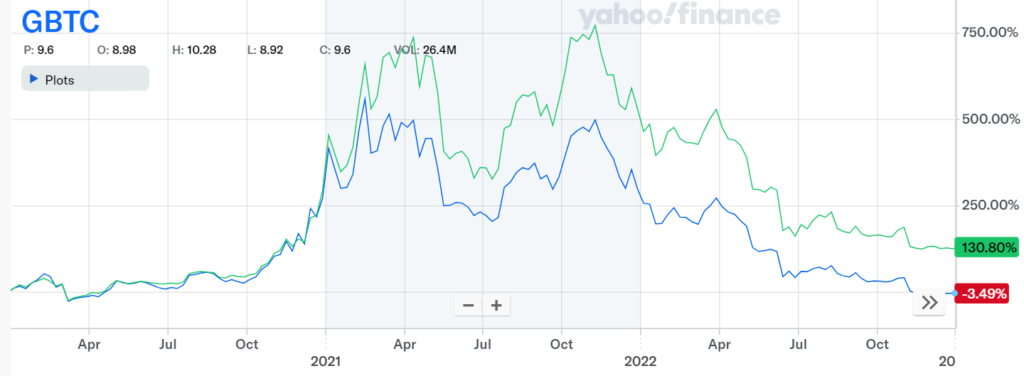
What do you notice? At the beginning of 2021, while BTC kept rising, many investors started to cash out and sell GBTC creating a gap and effectively inverting the “arbitrage” upside down. At that point, 3AC positions started to go underwater and the risk they overlooked before will now cost them dearly: GBTC was a closed trust, meaning they could not reclaim the BTC from it giving back the units. If GBTC was an ETF like it is today, maybe 3AC could have survived, but unfortunately, it wasn’t.
4 – At this point, 3AC not only started to lose a lot of money on this trade, but when in October 2021 the BTC big sell-off began, their lenders holding GBTC collateral started to issue margin calls the more the price was going down. What did 3AC do in this situation? They started to borrow even more to meet the margin calls and avoid being liquidated because at that point their “paper losses” would have become even more real.
5 – 3AC had a strong reputation in the market still at this point, so new lenders did not bother to do any due diligence on them.
6 – The situation precipitated once 3AC’s LUNA trades started to collapse as well, but there is no need to complicate things at this point.
If the Synnax protocol had already been in place in 2021, lenders would have immediately noticed from the ML models’ predictive outputs the growing difference between the NAV the firm claimed and where the data pointed instead. The beauty of machine learning models is that they cannot only analyze the given training data but also learn trends and patterns that can be identified in unseen data to provide an accurate prediction at inference. In 3AC’s case, the models would have surely picked up the correlations between 3AC’s assets and liabilities and GBTC price, BTC price, and the GBTC/BTC premium ultimately projecting NAV values much different from those the company was sharing as we saw above.
At this point, you might ask, what if 3AC wasn’t being honest and sharing all its data? Synnax’s “ZK/e” score, which stands for “Zero-Knowledge Proof of Equity”, would have reflected a low transparency score ultimately factored into the probabilities of default calculations related to 3AC (the lower the ZK/e score the higher the impact of the poor “data quality” discount into the ML models calculation consequently resulting in a higher probability of default metric).
What about “traditional” companies (i.e. Web2)? The Synnax protocol is likewise very effective because, as I mentioned at the very beginning, models only care about data and are agnostic to all those factors human judgment tends to build in Credit Analysis considerations more often than not including biases that will ultimately lead to mistakes, overlooking risks, and then losses.